BEFORE the 20th Century there was no age for retirement. There existed a leisured class who through birth or industry could choose what work they did – if and when. But, even they didn’t retire.
Retirement, like unemployment, can potentially reduce you to discussion of people, events, and lost opportunities, when great minds discuss ideas. Of course, even greater minds discuss numbers and ideas.
So if you are keen for a sea change, and are a graduate from a science or engineering discipline who enjoys problem solving, consider moving to Noosa and enrolling in a PhD or masters in weather and climate forecasting using artificial intelligence.
Applicants must be Australian citizens or permanent residents or New Zealand citizens, and must be enrolled or intending to enroll in an eligible research higher degree program at CQ University, and be based at the Noosa campus. It is expected that applicants will like problem-solving and playing with numbers; have an ability to work independently, but also be able to follow directions; and want to build a portfolio of co-authored peer-reviewed publications.
The successful applicants will each be provided with a tax-exempt living allowance scholarship for a fixed term of up to 3.5 years, with a commencing stipend of $32,000 per annum.
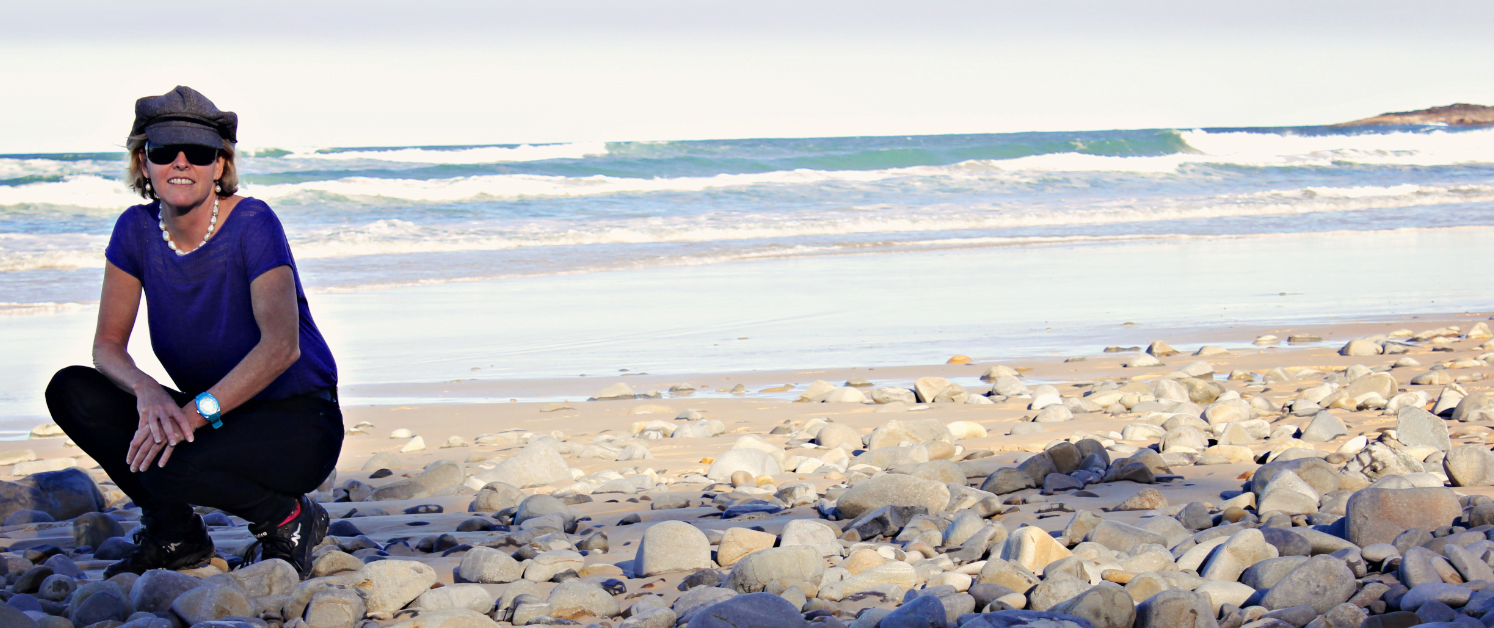
POSSIBLE projects include, but are not limited to, the following:
1. Forecasting El Niño-Southern Oscillation
For three decades, there has been a significant global effort to improve El Niño-Southern Oscillation (ENSO) forecasts with the focus on using fully physical ocean-atmospheric coupled general circulation models. Despite the increasing sophistication of these models, their predictive skill remains only comparable with relatively simple statistical models, with some blaming a phenomenon known as the Spring Predictability Barrier (SPB). Preliminary studies suggest that artificial neural networks can forecast through the SPB. It is possible further advances could be made through the refining of input variables building on the work of Aiming Wu (see Neural Networks, Volume 19), and possibly by also potentially considering extra-terrestrial influences including atmospheric tides (see Ken Ring, The Lunar Code).
The development of an improved method for forecasting ENSO through the elucidation of the most relevant input variables could be the focus of this project.
2. Signal processing to understand drivers of rainfall
There is a natural relationship between artificial neutral networks and signal processing. The neural network software that underpins our current prototype models was developed at the University of Florida by researchers in their department of electrical engineering with expertise in signal processing. Our prototype models, however, do not explicitly decompose the rainfall time-series signals into components. If the component signals were elucidated it would potentially aid understanding of the drivers of rainfall, and potentially improve forecasts.
Exploration of these concepts could form the central theme of a project that would best suite a graduate with a background in signal processing and/or electrical engineering.
3. Considering cyclical changes at the Antarctic to forecast rainfall in the Murray Darling
Australian farmers have long sought advice from long-range weather forecasters who operate independently of the Bureau of Meteorology, perhaps beginning with the work of astronomer Inigo Owen Jones. Modern forecasters using the same cyclical variations claims a strong relationship between higher sea ice averages in the Antarctic and periods of below average rainfall for eastern Australia and heavier late season frosts (see Kevin Long, www.thelongview.com.au). The Antarctic Oscillation (also known as the Southern Annular Mode or SAM) is also thought to be an important driver of rainfall variability in southern Australia (see Australian Bureau of Meteorology, http://www.bom.gov.au/climate/enso/history/ln-2010-12/SAM-what.shtml).
The focus of this project could be input selection and optimisation for monthly rainfall forecasting in the Murray Darling, including a consideration of the Antarctic Oscillation and changes in sea ice extent.
4. Modelling past temperatures and forecasting future temperatures – globally and locally
General circulation models, that underpin the current dominant paradigm in climate science and forecast global warming, simulate climate based on an assumed first principles understanding of the physical process. In contrast, ANNs rely on historical climate data to acquire knowledge, learn relationships, model and measure relationships and then use this information to make forecasts.
ANNs could be used to both provide an independent forecast of future temperatures, and as an independent method of GCM validation under future climate. Limited research is already occurring in this area (e.g. Kisi and Shiri, International Journal of Climatology Volume 34) and could be the focus of more than one PhD and/or Masters project. Such projects could also explore local, regional and global variability in temperatures historically and into the future.
The integrity of historical temperature data is largely irrelevant to the performance of a GCM, but critical to the operation of an ANN. So projects that focused on the use of ANN for forecasting future climate, would very likely benefit from first developing a technique for creating continuous series of high quality temperature data for individual locations as an input variable. While such temperature series theoretically already exist, they are not stable over time and often represent a modelled version of the temperatures originally recorded (see Zhang et al, Theoretical and Applied Climatology, Volume 115; Stockwell and Stewart, Energy & Environment, Volume 23; J. Nova http://joannenova.com.au/tag/homogenization-temperature-data/ T. Heller http://stevengoddard.wordpress.com/2014/07/18/nasa-hacking-australia/; B. Dedekind http://wattsupwiththat.com/2014/06/10/why-automatic-temperature-adjustments-dont-work/; Marohasy et al., The Sydney Papers Online, Issue 26).
5. Forecasting rainfall to aid mine scheduling
There is a need for more skillful medium-term rainfall forecasts for the Bowen Basin, a key coal-mining region in Queensland. Official seasonal forecasts are currently based on general circulation models, are not reliable, and do not provide adequate information in terms of timing and strength of rainfall for mine scheduling and pro-active risk management. V.S. Sharma and colleagues detail these issues in a report published by the National Climate Change Adaptation Research Facility in 2012.
The focus of a PhD or masters could include investigation of the possibility of using ANNs to generate forecasts for shorter time intervals (2 weeks and 1 week) and shorter lead times (2 weeks and 1 week) and using humidity, atmospheric pressure, cloudiness, wind direction and speed, as well as key climate indices as input variables.
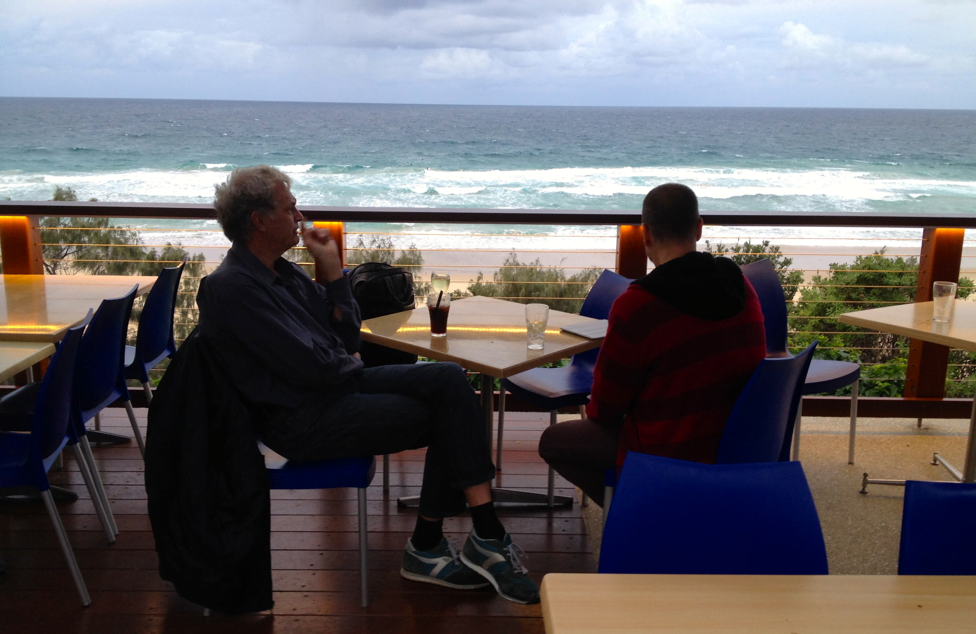
NEXT STEP, if you are interesting in applying, or just want more information, please contact me on mobile 041 887 32 22 or email jennifermarohasy at gmail.com. Closing date for applications is 30th October 2014.
THERE is more information on the scholarships at the CQ University website at:
http://www.cqu.edu.au/research/future-candidates/scholarships
General information about ANNs is taught as part of machine learning courses. Yaser Abu-Mostafa at the California Institute of Technology offers such an introductory online course, which includes some theory, algorithms and applications, available for download and viewing at https://work.caltech.edu/telecourse.html.
Our ANNs are based on software developed by Neurosolutions. More information on this software is available at http://www.neurosolutions.com .
Recent relevant publications by John Abbot and me include:
Abbot J., Marohasy J., 2015. Using artificial intelligence to forecast monthly rainfall under present and future climates for the Bowen Basin, Queensland, Australia. International Journal of Sustainable Development and Planning. In press
Abbot J., Marohasy J., 2014. Input selection and optimization for monthly rainfall forecasting in Queensland, Australia, using artificial neural networks. Atmospheric Research 128 (3), 166-178
Abbot J., Marohasy J., 2013. The potential benefits of using artificial intelligence for monthly rainfall forecasting for the Bowen Basin, Queensland, Australia, In: Brebbia, C.A. (Ed.), Water Resources Management VII, WIT Press, Southhampton, (on-line) doi:10.2495/WRM130261
Abbot J., Marohasy J., 2012. Application of Artificial Neural Networks to rainfall forecasting in Queensland, Australia. Advances in Atmospheric Science 29, 717-730
Relevant other references include:
Australian Bureau of Meteorology, 2014. The Southern Annular Mode (SAM) http://www.bom.gov.au/climate/enso/history/ln-2010-12/SAM-what.shtml
Dedekind, B. 2014. Why automatic temperature adjustments don’t work http://wattsupwiththat.com/2014/06/10/why-automatic-temperature-adjustments-dont-work/
Heller A., 2014. NASA Hacking Australia http://stevengoddard.wordpress.com/2014/07/18/nasa-hacking-australia/
Halide H., Ridd P., 2008. Complicated ENSO models do not significantly outperform very simple ENSO models. International Journal of Climatology 28, 219–233
Kisi O., Shiri J., 2014. Prediction of long-term monthly air temperatures using geographical inputs. International Journal of Climatology 34, 179-186
Long K., 2014. Current forecasts http://www.thelongview.com.au/forecast.html
Marohasy J., Abbot J., Stewart K., Jensen D., 2014. Modelling Australian and Global Temperatures: What’s Wrong? Bourke and Amberley as Case Studies. The Sydney Papers Online, Issue 26. http://www.thesydneyinstitute.com.au/paper/modelling-global-temperatures-whats-wrong-bourke-amberley-as-case-studies/
Ring K., 2006. The Lunar Code. Random House, New Zealand, pp 208
Risbey J. S., 2009. On the remote drivers of rainfall variability in Australia. Monthly Weather Review 137, 3233-3253
Sharma V.S, et al. 2012. Extractive resource development in a changing climate: Learning the lessons from extreme weather events in Queensland, Australia, National Climate Change Adaptation Research Facility, Gold Coast, pp. 110.
Stockwell D., Stewart K, 2012. Biases in the Australian High Quality Temperature Network, Energy & Environment, Vol. 23, 10.1260/0958-305X.23.8.1273
Wu A., Hsieh W.W., Tang B., 2006. Neural network forecasts of the tropical Pacific sea surface temperatures. Neural Networks 19, 145–154
Zhang L. et al. 2014. Effect of data homogenization on estimate of temperature trend: a case of Huairou station in Beijing Municipality. Theoretical and Applied Climatology 115, 365-373
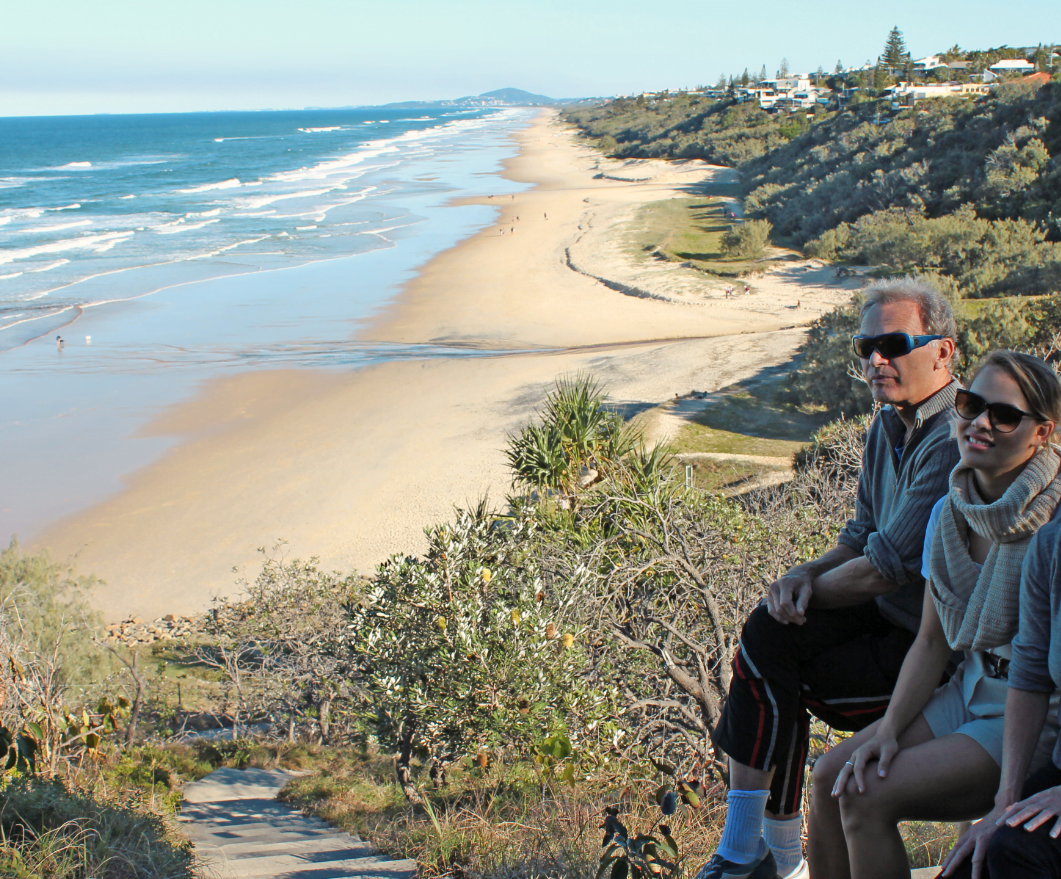
Wow, just wow!
I was there just last weekend. Noosa is a beautiful part of the world.
Good for you Jen.
Reading about your latest coup piqued my curiosity about CQ University. My general impression from the Wiki article is that CQU is an innovative institution, and probably qualifies as the ‘Skunkworks’ of government-funded higher education in Australia.
http://www.lockheedmartin.com/us/aeronautics/skunkworks.html
I immediately thought of my friend Mamikon Mnatsakanian, who is an astrophysicist, and one of the world’s leading mathematicians.
http://en.wikipedia.org/wiki/Mamikon_Mnatsakanian
More than a decade ago, Mamikon created a job for himself, working with Professor emeritus Tom Apostol, of Caltech. Together, they have created a record for the greatest number of publications in the shortest amount of time, at American Mathematical Monthly.
http://en.wikipedia.org/wiki/American_Mathematical_Monthly
Mamikon has created a new branch of maths, which he calls Cakulus. The special thing about Cake-ulus (that’s how it’s pronounced) is that it’s the only mathematical subject, which *increases* the self-esteem of those who study it, rather than convincing people that they’re stupid.
Cakulus is a visual approach for finding areas and volumes of some classical geometric figures, for which Integral Calculus is traditionally used. In fact, the area of a Bicyclix is unsolvable with standard calculus, but with Cakulus, a 12-year old child can easily understand it. There are no formulas to memorize, and no equations to solve with Cakulus. Instead, one draws pictures that lead to an Aha experience. Mamikon’s favorite metaphor is slicing a cake; hence the name of the subject.
Mamikon has taught Cakulus — plus mathematical games and puzzles for enrichment — to local Montessori kids, and they loved it. They called him Magic Mamikon. He was eventually able to teach a Cakulus course at University of California at Davis. However he had to overcome a lot of resistance from the Mathematics Department. Talk about paradigm fixation!
Anyway, it strikes me that Mathematics for Self-Esteem would be CQU’s cup of tea. These days, Mamikon’s health is very fragile, and I doubt that he would be up for a visit to Australia. But no worries! Videos could fill the gap.
I don’t know if Mamikon made any videos of his earlier courses. If not, he’d need to find an audience for a Cakulus course at a local high school or junior college, and make the videos from scratch.
The person who teaches/facilitates Cakulus at CQU need not be a mathematician. Because of the paradigm issue that I mentioned earlier, an engineer would probably do a better job.
What do you think?
Hey Larry
Thanks for your enthusiasm! I’m also inclined to think “wow”… what an opportunity… for me and also for several science and/or engineering graduates who may be wise enough to know the joys of being able to pursue an interest/to solve a puzzle, and one that potentially has broad application.
Your friend Mamikon A. Mnatsakanian, the astrophysicist, sounds like a clever and interesting fellow! But rather than teach calculus, I would like him to come to Noosa and think about how to quantify the effects of the moon and planets on earth’s weather and climate.
The first of the possible projects listed above makes reference to atmospheric tides. We know the moon has a significant gravitational effect on the earth, and its declination broadly mirrors the timing of ENSO events, but how can this be effectively quantified for input into an ANN?
Artificial neural networks (ANN) need data… an astrophysicist who is creative is just the person we need. To identify and then describe the extraterrestrial phenomena in terms of series of numbers that can be feed into an ANN.
Hey Debbie
Next time your in Noosa you MUST phone, we could have caught-up for lunch or dinner, or better still, a walk in the National Park… it is a little cold for swimming right now.
Jennifer,
When he lived in the old Soviet Union, Mamikon did some mathematical modelling: seismic safety of kindergarten classrooms, and of a nuclear power plant in his native Armenia. His tentative results were very unPC. He was very in-your-face about his findings. And he made a number of political enemies.
Mamikon made a trip to America to raise funds from Armenian-Americans for computer hardware for his modelling projects. Then the USSR suddenly collapsed, and he found himself in an ambiguous situation with respect to his visa. He was stranded in a country whose language he did not speak, and he was officially a citizen of a country that no longer existed!
If Mamikon were to take part in your ANN projects, he would need to quick-study some geo-physics. And there’s always his health situation.
My understanding is that Mamikon’s first loyalty is to his boss, Tom Apostol. The publications are mostly Mamikon’s inspirations. Tom critiques and fine-tunes them. Then Tom does the actual write-ups.
English is Mamikon’s third language, after Armenian and Russian. Unlike most people, Mamikon loves public speaking. And he would rather do the maths, draw diagrams, and write computer programs; than compose pretty purple prose — even in Russian.
Mamikon is following his mathematical dreams, but his physics dreams are on hold.
Tom is getting long in the tooth. And Mamikon may be looking for a Plan B for the future. Another possibility: Mamikon’s participation in your ANN projects could be part-time and online, until he finds himself unemployed if something happens to Tom.
Of course, I cannot speak for Mamikon, and cannot ‘volunteer’ him for anything. On the other hand, I don’t think that he plans to retire — ever. And even if his plate is full, he would appreciate having a friend in Australia. If you contact him, please mention that I brought up the subject.
So, I’ve had some emails challenging me over the value of using artificial neural networks (ANNs) for weather and climate forecasting.
Disappointingly most of these emails suggest a basic misunderstanding of what ANNs are. ANNs represent a form of machine learning, and this technology is applicable where you have 1. data, 2. patterns/signals embedded in that data, and 3. relationships that can not be easily reduced to simple formulae.
The problem of forecasting weather and climate fits all three categories: 1. there is so much data available, 2. cycles of heating and warming and/or wet and dry, can be seen within much of this data whether it relates to temperatures across the Pacific or rainfall at point locations, and 3. preliminary work by John Abbot and myself shows how ANNs can use this information to make forecasts about rainfall and also ENSO for some months into the future.
While many tell me that its impossible to forecast such chaotic systems for any period into the future, I would suggest that the proof is in the pudding, in the evidence that we can get reasonable skill scores from our prototype models. So much more could be achieved if we had a team.
If you are interested in learning more about ANNs, consider downloading an online course by Yaser Abu-Mostafa from Caltech that is available here: https://work.caltech.edu/telecourse.html . It provides background into the technology that we use. But the PhDs and masters are about the application of this technology for weather and climate forecasting, rather than creating it.
If you are interested in some of the related philosophical arguments including about the future of anthropogenic global warming, consider reading a recent blog post by myself with some insight comments in the thread that follows: http://jennifermarohasy.com/2014/07/three-facts-most-sceptics-dont-seem-to-understand/
Jennifer,
You wrote: “While many tell me that its impossible to forecast such a chaotic systems for any period into the future, I would suggest that the proof is in the pudding, in the evidence that we can get reasonable skill scores from our prototype models.”
I’d like to give a long-winded, philosophical elaboration on the point that you just made. In the physical science sense, “chaos” means: sensitive dependence on initial conditions (SDOIC). A system can be chaotic even when we have a *perfect* understanding of how it works! How so?
Suppose that weather qualifies as a chaotic system. Suppose futher that we have a perfect understanding of *all* of the underlying mechanisms. (This is clearly not the case.)
Then the accuracy of medium-term forecasts for a hypothetical perfectly understood weather system, which encompasses our entire fair planet, is limited by measurement uncertainty. *All* measurements — in contrast with counting — have uncertainty. This generalization applies to temperature measurements, to precip measurements, and to insolation measurements, among other things.
Meteorological measurement error is an even bigger issue for large areas, because the best that we can do is to make a planet-wide grid, and to take large numbers of measurements — at 1 km intervals, for example.
After taking the first round of measurements, we feed them into our super-duper computer model. The errors from Round 1 of the measurements will inevitably introduce discrepancies between predicted values and the actual measurements in Round 2. Propagation of error between successive rounds will lead to an increasingly large divergence in the intermediate term. Iteration error, anyone?
Computer simulations suggest that a chaotic system can temporarily settle into an apparent pattern. This is called an attractor. Then the system can abruptly break out of the apparent pattern, and settle into a new temporary pattern. Attractors can be a bête noire for investigators with subconscious confirmation biases.
Inperfect understanding, together with the perception of chaos, is why TV weather people in Northern California usually limit themselves to 5-day forecasts. They have no desire to become laughingstocks. So much for chaos theory, as applied to weather forecasting.
Now here’s the kicker: Can anyone prove that weather is ultimately chaotic? It may very well be that phenomena, which we don’t understand very well at the moment — like changes in sunspot activity, and in the Earth’s magnetic field — may play important roles in weather changes in the intermediate term. And in the long term.
The SDIOC hypothesis, as applied to medium-term weather forecasting, is not falsifiable. The assumption that SDOIC is *the* limiting factor in medium-term weather forecasting is an article of faith; there is no evidence for that proposition. In general, throwing up one’s hands and invoking the Gods of Chaos, is a cop-out.
Here’s my response to Ned Naysayer: The monkey is on your back. If you have no evidence for your belief that SDIOC is the ultimate barrier to medium-term weather forecasting, then get out of the way. Let real scientists, with the help of ANNs, learn more about the interwoven control variables of the planetary weather system.
Yes, chaos exists. So what?
The critical question is whether machine learning can be implemented to provide superior predictions when compared to other available methods.
The published results show quantitatively that machine leaning produces superior results for prediction of medium term rainfall in Queensland when compared to GCMs.
Therefore the machine leaning techniques, particularly neural networks, have practical utility as a rainfall forecasting method, and are a lot cheaper to implement.
Well said Larry!
From my perspective as a producer who gambles with the weather/climate year in and year out – I think anyone who is willing to improve regional seasonal forecasting skill – deserves encouragement.
Good for you Jen.
Very strange that you are being challenged before you have even formed your team.
Obviously the funding is already organised & it’s
presumably not a bottomless taxpayer pit, so therefore not particularly threatening anyone?
Larry’s right. . . if they are just being sniping and overly critical/cautious naysayers with no proof that it can’t be done. . . they would do better to
stay out of the way.
If you & your team are successful in improving forecasting skill via ANNs that would be a highly useful and valuable outcome.
Hey Debbie,
The funding for the scholarships is being provided by the B. Macfie Family Foundation. Bryant Macfie is a Perth-based, retired medical doctor keen to support evidence based scientific research.
Successful applicants get $32,000 per annum tax free. It is not a lot of money, but if you already have some savings, and are more interested in ideas than money.
Congratulations Jennifer and John. Great achievement.
I’m a music, sound and acoustics person, as you know, so I tend to see things in terms of vibrations and frequencies and cycles. Everything we hear is a series of cycles, everything we see is a series of cycles, in fact everything is a series of cycles all interacting with each other just as the sounds of a music chord react with each other.
To find those frequencies and interactions – the chords – in weather cycles is an idea I can relate to.
Jen has always suggested that weather is not necessarily chaotic and maybe it’s not if you understand the cycles.
Wow, this is an amazing thing you have put together. Most impressive. I am going to refer a friend of mine from Melbourne to have a look at this. He may have some time on his hand, and he certainly has the tech credentials.
Actually I just remembered a young Australian pal of my son I met when visiting my son in the SF Bay Area. So I will forward this to two possibly qualified Australians.
Best wishes. If I can do more I would be pleased to do so.
As someone very wise once said, (paraphrasing) “bad paradigms don’t die, they get replaced with better ones.”
Good luck on the hunt.
Much thanks, Hunter! If people could circulate this information, there is a chance, together, we could find some great minds to help build a new approach with new tools.
Can I throw in a couple of really wild left field ideas, just in case they spark something.They’re “models” of natural cycles from sources not usually thought of as that.
Calendars and festival dates developed a couple of thousand years ago by various peoples around the world may contain clues about cyclic seasonal trends in their region.
Polynesian navigation stick charts –
http://thenonist.com/index.php/thenonist/permalink/stick_charts/
http://en.wikipedia.org/wiki/Marshall_Islands_stick_chart
They represent disturbances in ocean swells and currents, so are actually maps of turbulence on a really large scale.