THE accurate weather forecast of James Stagg back in June 1944 is considered crucial to the successful D-day invasion that changed the course of WWII.
Should we find ourselves at war again, is there an institution or individual capable of providing an accurate short or long-term weather forecast?
Dr John Abbot and I are working towards better medium-term rainfall forecasts, as explained in the highlights to our latest research paper:
1.Monthly rainfall forecasts for agricultural areas in Queensland and Western Australia,
2. Forecasts more skilful than produced by Australian Bureau of Meterology using General Circulation Models, and
3. Potential to provide better warnings of extreme flooding events with long lead times.
This paper is provided free by scientific publishers Elsevier for a limited period (50 days) at: https://authors.elsevier.com/a
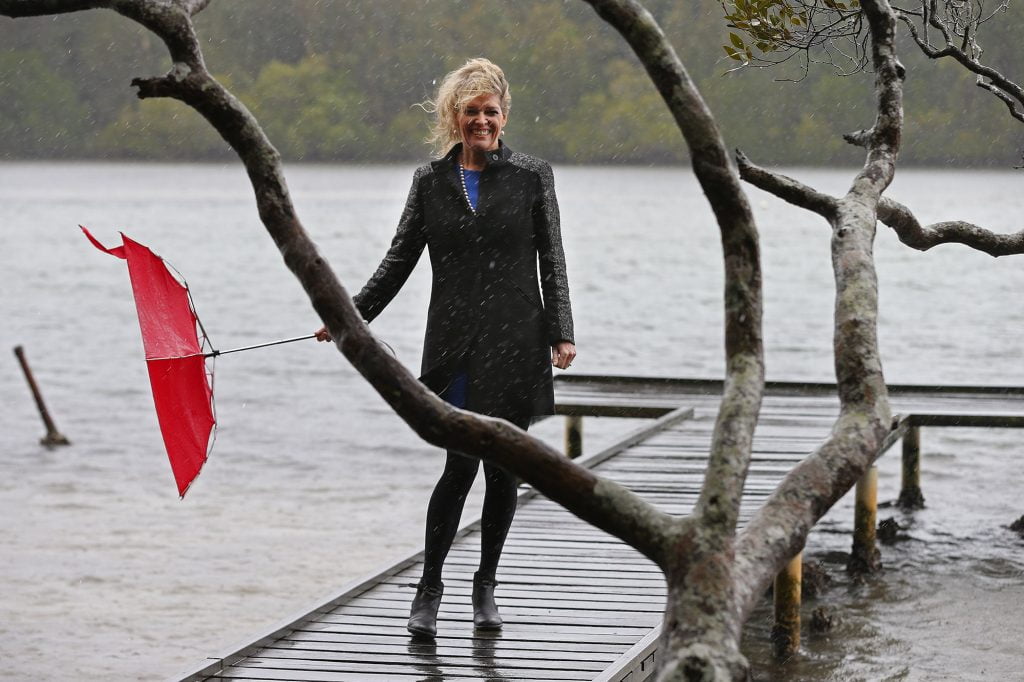
Hi Jennifer,
I have just download your paper and I have a couple of observations.
The neural network approach appears to be impressive and seems to works well for individual locations. Have you tried using it to model and predict rainfall at a regional level? The BOM normally gives predictions for regions I believe and not for specific locations , so your results may not be comparable
A neural network approach will give results that are tailored for each location and I think that is why you appear to get such impressive results. The problem may be that what suits one region does not necessarily suit another location. What is the spatial correlation for your fitted model and parameters for nearby locations?
To emphasize. neural networks with multiple models and dozens of parameters are almost guaranteed to work and this is both their strengths and weaknesses. As per John Von Neumann’s famous quote “With four parameters I can fit an elephant, and with five I can make him wiggle his trunk.” As a reminder, John Von Neumann pioneered the theoretical development of neural networks.
In this context You can get impressive results for particular cases (in this case locations) . What would be more convincing would be to test your model(s) against future prediction for these locations. It seems sensible to use your model to predict the next 12 months rainfall from today with ,say monthly resolution, at each location and compare these predictions with the climatogical averages. We could then test your predictions and see how well they perform. Maybe you could post your predictions here?
However in reality the pertinent question is can you do better than the BOM on a regional basis rather than at specific locations? I would be really impressed if your modelling gives a significant improvement over the BOM ‘s model on this basis.
I have one other significant comment in that the 3rd term in equation (1) in your paper seems to be inappropriate. You are using the Mean Absolute Error (MAE) rather than the Mean Bias Error(MBE). Firstly the the third term MAE and and the first term Roor Mean Square Error (RMSE) are often significantly correlated (particularly if outliers are infrequent) so instead of fitting 3 parameters you are essentially fitting 2 (and maybe a bit) parameters.
Dawson’s paper (https://www.hydrol-earth-syst-sci.net/16/3049/2012/hess-16-3049-2012.pdf) states sensibly that identification and removal of highly correlated metrics is recommended (section 2.30 – metric orthogonality) .
I think the confusion may have arisen from the definition Jennifer has used comes from the paper by Malamos and Koutsoyiannis (http://geo.teimes.gr/web/nm-en/wp-content/papercite-data/pdf/malamos2015d.pdf).
These authors have used ME in their equation for IPE3 which unfortunately Jennifer and her co-author have misinterpreted as the Mean Absolute Error (MAE) instead of Mean Bias Error (MBE) -see equation A6 in the Appendix. It is clear from the line immediately preceding this equation that it was clearly meant to refer to MBE.
Actually I would have thought that the MBE (rather than the partially redundant MAE) would have been a key parameter to fit and you would want to get that right. Unfortunately this does not appear to be the case.
I am not sure how this got through peer review but someone who is asked to review a paper is supposed to actually read the references provided in the paper!, Maybe it’s a mistaken belief and I have been doing it all wrong- what a waste of time and effort!
One other thing to note is that Jennifer or her collaborator have lifted segments of Dawson’s paper (changing one or two words here or there). Compare the description of IPE above with section 2.1 in Dawson et al.
Apropos of the above, I would like to thank Jennifer, yet again, for not censoring my latest contribution.
I believe that Jennifer is a staunch proponent of free speech (like her colleagues at the API). I would hate to be sent to Coventry again (despite the pleasant weather at this time of the year) .
Thank you for the update and congratulations on your progress. Far too many involved with weather and climate work are grubbing along in the climate hype derivative echo chamber. On the other hand, you and your colleagues are actually working on something that impacts the quality of life for many.
Dear Jennifer,
We would like to thank you for referencing our work in your publication: Skilful Monthly Rainfall Forecasts.
After reading the comments made by “MikeR” about the use of IPE, I have to clarify that the metrics used for IPE calculation in Malamos & Koutsoyiannis, 2016 are: Mean Bias Error (MBE), Root Mean Square Error (RMSE) and the coefficient of determination (R2), as clearly stated in the appendix text of the paper and proposed by Domínguez et al., 2011.
On the other hand, I am thinking that this could be a typical case of mistyping equation (1) in your manuscript.
Sincerely,
Nikolaos Malamos
References
Domínguez, E., Dawson, C. W., Ramírez, A. & Abrahart, R. J. (2011) The search for orthogonal hydrological modelling metrics: a case study of 20 monitoring stations in Colombia. J. Hydroinformatics 13, 429. doi:10.2166/hydro.2010.116
Malamos, N. & Koutsoyiannis, D. (2016) Bilinear surface smoothing for spatial interpolation with optional incorporation of an explanatory variable. Part 2: Application to synthesized and rainfall data. Hydrol. Sci. J. 61(3), 527–540. doi:10.1080/02626667.2015.1080826
Quite clearly, looking at the skill scores, the neural network approach is far superior to that used by the BOM with its GCMs.
The BOM method generates skill scores for monthly rainfall forecasts that are about the same as climatology. They spend millions of dollars to generate forecasts equivalent to taking an average of 30 numbers. A competent 10 year schoolboy could do this without even using a calculator.
Time for the BOM to enter the modern world of artificial intelligence and machine learning and provide the public with something useful.
Neville did you read my comments above? Clearly not. Jennifer’s grandiose claim that she outperforms the BOM is unjustified. She is predicting rainfall for a few specific locations while the BOM makes predictions on a regional basis.
As I explained, Jennifer’s approach is guaranteed to produce an almost perfect result due to the number of input parameters (120) , some of which are culled down by the software. Jennifer neglects to mention which ones survive and whether the survivors are consistent between different sites. Reporting these details in a paper would of course be sensible, which is not Jennifer’s strong suit.
To settle this I suggest Jennifer uses her neural network (using sensible metrics this time) to forecast rainfall, starting with this coming spring for Victoria, NSW etc. and compare her results with the BOM’s predictions.
Looking at the Neural Network software she is using, it looks particularly easy to feed the network with the appropriate data so I am not sure what has stopped her modelling regions. I might download the trial version and have a go myself.
With regard to the Malamas comment, it is good that he admits that his equation(1) had a simple typo-logical error . This had the unfortunate effect of confusing Jennifer and her colleague so that the wrong parameter was used in Jennifer’s paper. Jennifer’s mistake was repeated three times in her paper, even explicitly identifying the parameter as Mean Absolute Error instead of Mean Bias Error in Section 2 ( just above Table 4) .
Her use was also totally inconsistent with the definitions in Dawson’s paper. To describe it as merely a typo would be inappropriate
.
So all in all it is pretty sloppy work that makes the BOM’s reputed 0.4C indiscretion at one or two sites on one or two days relatively insignificant